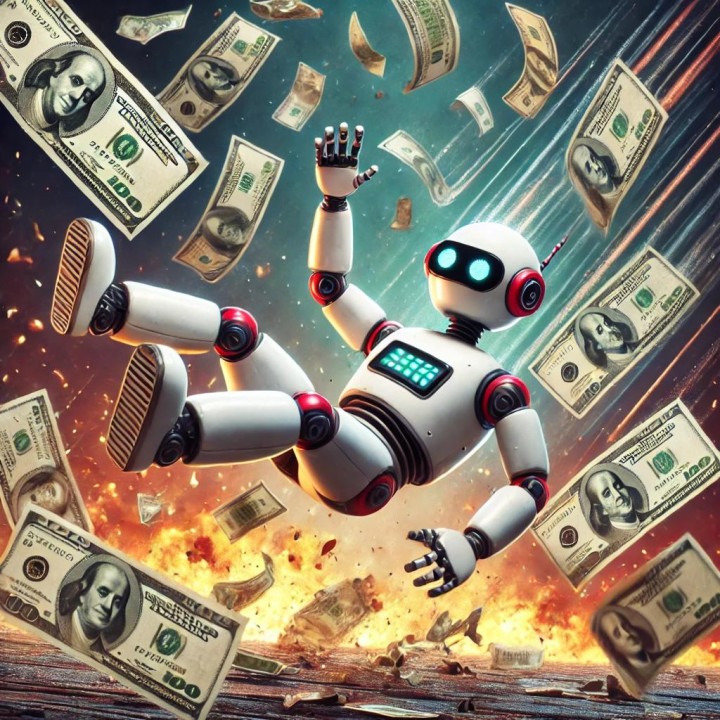
Overcoming the Generative AI Pitfalls: A Strategic Roadmap for Success
Amidst the generative AI hype, a sobering reality looms: Gartner predicts that 30% of these projects will be abandoned after proof-of-concept by the end of 2025. The reasons for this high failure rate are clear: poor data quality, inadequate risk controls, escalating costs, and unclear business value. However, by taking a strategic and informed approach, organizations can navigate these challenges and unlock the transformative power of generative AI.
Key Strategies for Generative AI Success:
- Strategic Alignment:Â Identify high-impact business use cases that align with your overarching strategy and priorities. Revisit previously deemed impossible opportunities and assess their feasibility with the latest generative AI capabilities.
- Dedicated Focus:Â Treat your generative AI project as a strategic initiative separate from your day-to-day operations. This will ensure the project receives the attention, resources, and focus it deserves.
- Data-Centric Approach:Â Evaluate your data’s quality from the outset and incorporate data cleansing and enrichment activities into your project plan. High-quality data is the foundation for successful generative AI models. Â
- Quantifiable Benefits:Â Clearly define and quantify your generative AI project’s tangible and intangible benefits. This will help you track progress and demonstrate the value of your investment to stakeholders.
- Robust Governance:Â Establish a robust governance framework for your generative AI project, similar to any other strategic investment. Assemble a team with the knowledge, experience, and skills to deliver on your project goals.
- Leverage Expertise:Â Partner with experienced third-party vendors who specialize in generative AI. Their expertise can help you avoid common pitfalls and accelerate your time to value.
- Start Small and Iterate:Â Begin with a pilot project to gain valuable insights and experience before scaling up. Embrace an iterative development approach, refining your model based on real-world feedback and results.
- Ethical AI:Â Prioritize ethical considerations in your generative AI development. Ensure your models are fair, unbiased, and transparent. Conduct regular audits to monitor for potential biases and address them promptly.
- Continuous Learning:Â Stay informed about the latest advancements and best practices in generative AI. The field is evolving rapidly, and ongoing learning is essential to remain competitive. Â
Conclusion:
Generative AI has the potential to transform industries and revolutionize the way we work. However, success requires a strategic approach prioritizing data quality, ethical considerations, and continuous learning. By following the roadmap outlined in this article and partnering with leading vendors like Palantir, organizations can overcome the common pitfalls and unlock the full potential of generative AI.
About the Author:
Harrison Lewis is the Founding Partner of Jacob Meadow and Associates, LLC, bringing over two decades of experience in digital transformation and IT innovation. With a distinguished career spanning leadership roles at Grocery Outlet, Northgate Gonzalez, HEB, and Kroger, Harrison has consistently driven success by implementing cutting-edge technology solutions. His expertise encompasses cloud adoption, SaaS, integration, and cybersecurity, ensuring clients receive tailored solutions that align with their business goals. Harrison empowers his team to deliver exceptional client results and is passionate about fostering a collaborative and innovative environment.